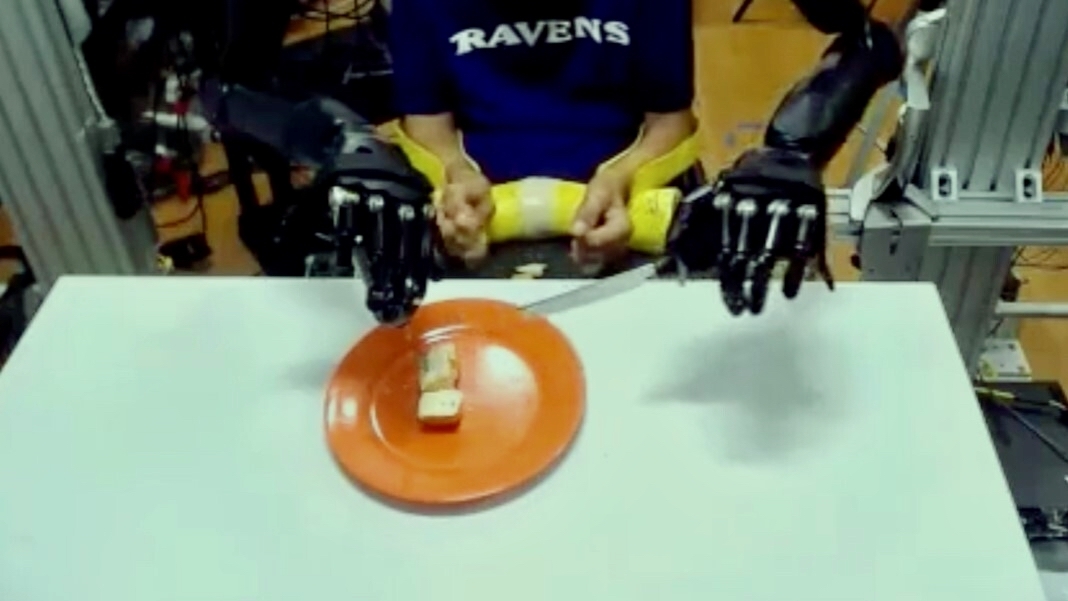
The man sat still in the chair, staring intently at a piece of cake on the table in front of him. Wires protruded from electrode implants in his brain. Flanking him were two giant robotic arms, each larger than his entire upper body. One held a knife, the other a fork.
“Cut and eat food. Move right hand forward to start,” ordered a robotic voice.
The man concentrated on moving his partially-paralyzed right arm forward. His wrist barely twitched, but the robotic right hand smoothly sailed forward, positioning the tip of the fork near the cake. Another slight movement of his left hand sent the knife forward.
Several commands later, the man happily opened his mouth and devoured the bite-sized treat, cut to personal preference with help from his robotic avatars. It had been roughly 30 years since he was able to feed himself.
Most of us don’t think twice about using our two arms simultaneously—eating with a knife and fork, opening a bottle, hugging a loved one, lounging on the couch operating a video game controller. Coordination comes naturally to our brains.
Yet reconstructing this effortless movement between two limbs has stymied brain-machine interface (BMI) experts for years. A main roadblock is the sheer level of complexity: in one estimate, using robotic limbs for everyday living tasks may require 34 degrees of freedom, challenging even the most sophisticated BMI setups.
A new study, led by Dr. Francesco V. Tenore at Johns Hopkins University, found a brilliant workaround. Robots have grown increasingly autonomous thanks to machine learning. Rather than treating robotic limbs as mere machinery, why not tap into their sophisticated programming so human and robot can share the controls?
“This shared control approach is intended to leverage the intrinsic capabilities of the brain-machine interface and the robotic system, creating a ‘best of both worlds’ environment where the user can personalize the behavior of a smart prosthesis,” said Dr. Francesco Tenore.
Like an automated flight system, this collaboration allows the human to “pilot” the robot by focusing only on the things that matter the most—in this case, how large to cut each bite of cake—while leaving more mundane operations to the semi-autonomous robot.
The hope is that these “neurorobotic systems”—a true mind-meld between the brain’s neural signals and a robot’s smart algorithms—can “improve user independence and functionality,” the team said.
Double Trouble
The brain sends electrical signals to our muscles to control movement and adjusts those instructions based on the feedback it receives—for example, those encoding for pressure or the position of a limb in space. Spinal cord injuries or other diseases that damage this signaling highway sever the brain’s command over muscles, leading to paralysis.
BMIs essentially build a bridge across the injured nervous system, allowing neural commands to flow through—whether it be to operate healthy limbs or attached prosthetics. From restoring handwriting and speech to perceiving stimulation and controlling robotic limbs, BMIs have paved the way towards restoring peoples’ lives.
Yet the tech has been plagued by a troubling hiccup: double control. So far, success in BMIs has largely been limited to moving a single limb—body or otherwise. Yet in everyday life, we need both arms for the simplest tasks—an overlooked superpower that scientists call “bimanual movements.”
Back in 2013, BMI pioneer Dr. Miguel Nicolelis at Duke University presented the first evidence that bimanual control with BMIs isn’t impossible. In two monkeys implanted with electrode microarrays, neural signals from roughly 500 neurons were sufficient to help the monkeys control two virtual arms using just their minds to solve a computerized task for a (literally) juicy reward. While a promising first step, experts at the time wondered whether the setup could work with more complex human activities.
Helping Hand
The new study took a different approach: collaborative shared control. The idea is simple. If using neural signals to control both robotic arms is too complex for brain implants alone, why not allow smart robotics to take off some of the processing load?
In practical terms, the robots are first pre-programmed for several simple movements, while leaving room for the human to control specifics based on their preference. It’s like a robot and human tandem bike ride: the machine pedals at varying speeds based on its algorithmic instructions while the man controls the handle bars and brakes.
To set up the system, the team first trained an algorithm to decode the volunteer’s mind. The 49-year-old man suffered from a spinal cord injury roughly 30 years before testing. He still had minimal movement in his shoulder and elbow and could extend his wrists. However, his brain had long lost control over his fingers, robbing him of any fine motor control.
The team first implanted six electrode microarrays into various parts of his cortex. On the left side of his brain—which controls his dominant side, the right-hand side—they inserted two arrays into the motor and sensory regions, respectively. The corresponding right brain regions—controlling his non-dominant hand—received one array each.
The team next instructed the man to perform a series of hand movements to the best of his ability. Each gesture—flexing a left or right wrist, opening or pinching the hand—was mapped to a movement direction. For example, flexing his right wrist while extending his left (and vice versa) corresponded to movement in horizontal directions; both hands open or pinching codes for vertical movement.
All the while, the team collected neural signals encoding each hand movement. The data were used to train an algorithm to decode the intended gesture and power the external pair of scifi robotic arms, with roughly 85 percent success.
Let Him Eat Cake
The robotic arms received some pretraining too. Using simulations, the team first gave the arms an idea of where the cake would be on the plate, where the plate would be set on the table, and approximately how far the cake would be from the participant’s mouth. They also fine-tuned the speed and range of movement of the robotic arms—after all, no one wants to see a giant robotic arm gripping with a pointy fork flying at your face with a dangling, mangled piece of cake.
In this setup, the participant could partially control the position and orientation of the arms, with up to two degrees of freedom on each side—for example, allowing him to move any arm left-right, forward-back, or roll left-right. Meanwhile, the robot took care of the rest of the movement complexities.
To further help the collaboration, a robot voice called out each step to help the team cut a piece of cake and bring it to the participant’s mouth.
The man had the first move. By concentrating on his right wrist movement, he positioned the right robotic hand towards the cake. The robot then took over, automatically moving the tip of the fork to the cake. The man could then decide the exact positioning of the fork using pre-trained neural controls.
Once set, the robot automatically moved the knife-wielding hand towards the left of the fork. The man again made adjustments to cut the cake to his desired size, before the robot automatically cut the cake and brought it to his mouth.
“Consuming the pastry was optional, but the participant elected to do so given that it was delicious,” the authors said.
The study had 37 trials, with the majority being calibration. Overall, the man used his mind to eat seven bites of cakes, all “reasonably sized” and without dropping any.
It’s certainly not a system coming to your home anytime soon. Based on a gigantic pair of DARPA-developed robotic arms, the setup requires extensive pre-programmed knowledge for the robot, which means it can only allow a single task at any given time. For now, the study is more of an exploratory proof of concept in how to blend neural signals with robot autonomy to further expand BMI capabilities.
But as prosthetics get increasingly smarter and more affordable, the team is looking ahead.
“The ultimate goal is adjustable autonomy that leverages whatever BMI signals are available to
their maximum effectiveness, enabling the human to control the few DOFs [degrees of freedom] that most directly impact the qualitative performance of a task while the robot takes care of the rest,” the team said. Future studies will explore—and push—the boundaries of these human-robot mindmelds.
Image Credit: Johns Hopkins Applied Physics Laboratory