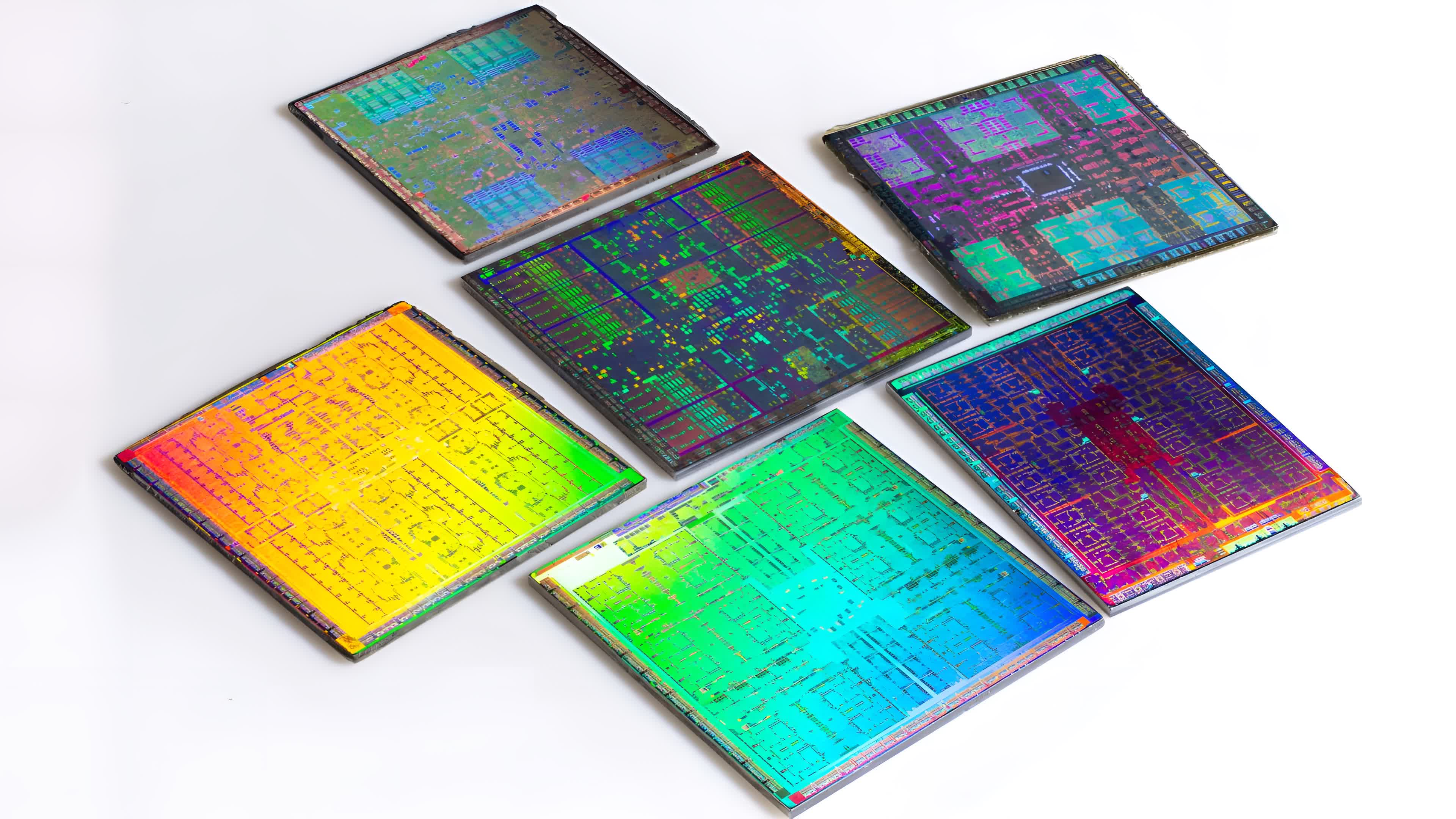
Forward-looking: When it isn’t busy building some of the most advanced silicon out there, Nvidia is exploring ways to improve the chip design process using the same silicon it’s making. The company expects the complexity of integrated circuit design to increase exponentially in the coming years, so adding in the power of GPU compute will soon turn from an intriguing lab experiment into a necessity for all chipmakers.
During a talk at this year’s GPU Technology Conference, Nvidia’s chief scientist and senior vice president of research, Bill Dally, talked a great deal about using GPUs to accelerate various stages of the design process behind modern GPUs and other SoCs. Nvidia believes that some tasks could be done better and much quicker using machine learning rather than humans doing by hand, freeing them to work on more advanced aspects of chip development.
Dally leads a team of around 300 researchers that tackle everything from the technological challenges around making ever faster GPUs to developing software that leverages the capabilities of those GPUs for automating and accelerating a variety of tasks that have traditionally been done mainly by hand. This research team is up from 175 people in 2019 and is set to grow in the coming years.
When it comes to speeding up chip design, Dally says Nvidia has identified four areas where leveraging machine learning techniques can significantly impact the typical development timetable. For instance, mapping where power is used in a GPU is an iterative process that takes three hours on a conventional CAD tool, but it only takes minutes using an AI model trained specifically for this task. Once taught, the model can shave the time down to seconds. Of course, AI models trade speed for accuracy. However, Dally says Nvidia’s tools already achieve 94 percent accuracy, which is still a respectable figure.
Circuit design is a labor-intensive process where engineers may need to change the layout several times after running simulations on partial designs. So training AI models to make accurate predictions on parasitics can help eliminate a lot of the manual work involved in making the minor adjustments needed for meeting the desired design specifications. Nvidia can leverage GPUs to predict parasitics utilizing graph neural networks.
Dally explains that one of the biggest challenges in designing modern chips is routing congestion — a defect in a particular circuit layout where the transistors and the many tiny wires that connect them are not optimally placed. This condition can lead to something akin to a traffic jam, but in this case, it’s bits instead of cars. Engineers can quickly identify problem areas and adjust their placing and routing accordingly by using a graph neural network.
In these scenarios, Nvidia is essentially trying to use AI to critique chip designs made by humans. Instead of embarking on a labor-intensive and computationally expensive process, engineers can create a surrogate model and quickly evaluate and iterate on it using AI. The company also wants to use AI to design the most basic features of the transistor logic used in GPUs and other advanced silicon.
Nvidia is taking the necessary steps to move to a more advanced manufacturing node, where many thousands of so-called standard cells must be modified according to complex design rules. A project called NVCell seeks to automate as much as possible of this process through an approach called reinforcement learning.
The trained AI model is used to correct design errors until it is completed. Nvidia claims that to date, it has achieved a success rate of 92 percent. In some cases, the AI-engineered cells were smaller than those made by humans. This breakthrough could help improve the design’s overall performance and reduce the chip size and power requirements.
Process technology is quickly approaching the theoretical limits of what we can do with silicon. At the same time, production costs rise with each node transition. So any slight improvement at the design stage can lead to better yields, especially if it reduces chip size. Nvidia outsources manufacturing to the likes of Samsung and TSMC. However, Dally says NVCell allows the company to use two GPUs to do the work of a team of ten engineers in a matter of days, leaving them to focus their attention on other areas.
Nvidia isn’t alone in going the AI route for designing chips. Google is also using machine learning to develop accelerators for AI tasks. The search giant found that AI can craft unexpected ways to optimize performance and power efficiency layouts. Samsung’s foundry division uses a Synopsys tool called DSO.ai, which other companies, big and small, are gradually adopting.
It’s also worth noting that foundries can also leverage AI manufacturing chips on mature process nodes (12 nm and larger) to address a lack of manufacturing capacity that has proven detrimental to the automotive industry’s operation over the past two years. Most manufacturers are reluctant to invest in this area, as the semiconductor space is highly competitive, focusing on the bleeding edge.
Well over 50 percent of all chips are designed on mature process nodes. International Data Corporation analysts expect this share to increase to 68 percent by 2025. Synopsis CEO Aart de Geus believes AI can help companies design smaller and more power-efficient chips where performance is not a top priority, such as cars, home appliances, and some industrial equipment. This approach is much less expensive than migrating to a more advanced process node. Additionally, fitting more chips on every wafer also leads to cost savings.
This story isn’t about AI replacing humans in the chip design process. Nvidia, Google, Samsung, and others have found that AI can augment humans and do the heavy lifting where increasingly complex designs are concerned. Humans still have to find the ideal problems to solve and decide which data helps validate their chip designs.
There’s a lot of debate around artificial general intelligence and when we might be able to create it. Still, all experts agree that the AI models we use today can barely deal with specific problems we know about and can describe. Even then, they may produce unexpected results that aren’t necessarily useful to the end goals.